advertisement
Here’s Why More African Enterprises Need Data Warehouses
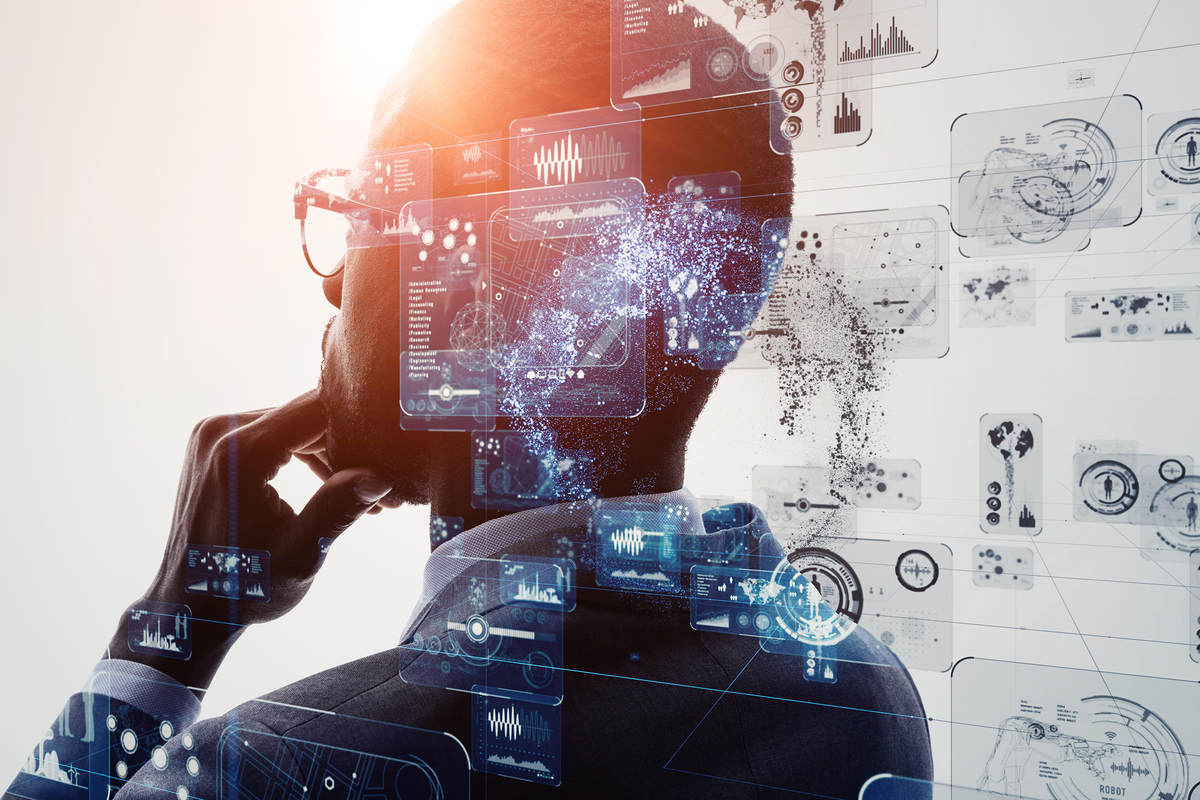
Like their global counterparts, African enterprises are churning out increasing volumes of data from every transaction and customer touchpoint, yet these troves of information are not being as well utilised as they could be, leading to lost opportunities for business intelligence.
One answer to this problem is the data warehouse, essentially a centralised data management system built for analytics and other business intelligence (BI) processes. But while enterprises in Africa and the Middle East are beginning to realise the importance of data collection and analysis, progress has been slow, according to the recent 2020 State of Data & Analytics MEA, report.
“Going back five years ago, data, being data-driven and especially analytics were kind of a ‘nice to have’ for companies,” Louise de Beer, Head of BI and Data Science at analytics-driven estate agent Leadhome said in the report. “Then, if you look at reports from two years ago, innovators started playing with data as a differentiator. I think where we are now is that data is becoming a hygiene factor. If you don’t have it, what are you doing with your business?”
advertisement
Nevertheless, business leaders are increasingly finding that breaking down silos and centralising data storage is a challenge, according to the report. The problem ranks second to the poor organisational understanding of the value of data, it stated.
Largely, the responsibility of data collection and analysis has fallen on CIO’s laps, though recently companies have been hiring chief data officers to specifically handle data management and warehousing. The increasing emphasis on data analysis is causing enterprises to take a fresh look at how they manage data.
What is a data warehouse?
Data warehouses are data management systems designed to store large volumes of data for analysis. Data warehouses may contain multiple databases, where data is ordered in tables and columns. The idea is that once data is organised this way, enterprises have quicker access to data, better query and system performance, and enhanced business intelligence.
advertisement
In a given enterprise there are numerous data collection points, whether via payments, email enquiries, sales and marketing, and various business units. At first glance, these data points might not be related, but put together and correlated, new insights can be realised.
Companies, though, need to have the data stored in one place so that it can be queried and reviewed for valuable insights. Data warehouses are where historical data is kept for later analysis.
“Data warehousing gives data engineers a single source of truth to query data for analysis purposes,” says Jeph Acheampong, a data engineer based in Ghana. He has trained data engineers across Africa through his education organisation, Blossom Academy.
advertisement
Large enterprises may opt to set up their internal data warehouse by configuring their systems and buying enterprise software to manage their data. This route has proved to be expensive but ensures that companies can protect their data according to the legal environment they operate in. Companies would then need to train the IT department on how the data should be stored, using a myriad of tools.
On-premises data management is usually the first step in integrating all the data collected in an organisation. A possible downside is that it could increase the company IT budget and alter workflows.
Data lakes vs data warehousing
Another industry-grade solution for storing big data is the data lake, and at a glance, it may seem similar to the data warehouse concept but in reality, the two types of systems are very different.
Data warehousing requires a process of cleaning up the data and having it structured before storing it. Data lakes, on the other hand, store data in their raw formats. These could be files, system logs, images, video, or text.
The flexible nature of data lakes makes the initial process of uploading data easier than what’s required for storage in a data warehouse. But data warehouses are particularly attractive for specific use cases, such as supporting reports or recurring dashboards that are consumed at a high frequency, according to Craig Kelly, VP of Analytics at Syntax, a managed cloud provider for enterprise applications.
Kelly intimated that it was easier to implement a data lake where analytics could be extracted later from the various forms of data. However, the structured storage format of data warehouses lends itself to fast queries and more immediate analysis for business intelligence.
Cloud-based data warehousing
The cost of owning and managing on-premise data software and hardware might scare away some companies seeking to dive into serious data analytics. Cloud-based data warehousing can ensure that there are no hardware costs in managing company and customer data.
Moreover, cloud-based data warehousing can be a solution for error proofing and redundancy of historical data when combined with on-premise solutions, Acheampong stated.
Cloud services can act as redundant storage in case there is a glitch in the company’s internal systems. Additionally, cloud-based solutions come with automatic updates for software ensuring smooth upgrades.
“Another essential benefit is the reduction in data management. When leveraging the cloud, the workforce needed to manage the data is reduced and rather deployed to working on other essential data projects,” Acheampong added.
Elasticity is a big factor to consider in cloud-based data warehousing. Depending on the data being churned, enterprises will have different needs at different times. Elasticity ensures that big data can be accommodated in the central repository.
How to implement data warehouses
Before a strategy to integrate data warehousing is implemented, an organisation needs to have a data management policy for all employees who are in contact with data.
Data policies ensure that employees do not tamper with information in the centralised repository and know how to save information in the correct format, Acheampong says. Customer privacy in dealing with information is also a key ingredient in the data management policy.
The next step is to put in place an extract, transform and load (ETL) process by using ETL tools such as SSIS (SQL Server Integration Services), Talend and Stitch, which pull data from different silos into one centralised system.
Cloud options such as BigQuery ETL and Amazon’s AWS Glue can also be implemented to support repositories to Google Cloud and Amazon respectively. Other third-party ETL tools work with other cloud providers.
Enterprises also should set up a database performance monitoring system, such as those offered by Solarwinds and Quest Software. This helps monitor the databases to ensure the integrity of the data coming in from the various sources.
“Additionally, companies often find it difficult to hire one person to monitor every database. (The performance monitoring) system also gives an overview of how the database is being utilised,” Acheampong explained.
He adds that the growth of data centres in Africa could also offer great help for organizations seeking to implement robust data warehousing strategies. These centres offer infrastructure, monitoring tools, failover clusters, and backup.
As the 2020 State of Data & Analytics MEA report states, it is up to organizations to restructure their operations alongside strong internal data analytics to get top data insights to drive their agenda with clarity.