advertisement
Data Problems Facing Africa’s AI Adoption
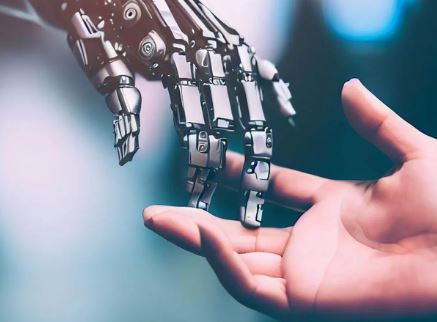
The increasing capabilities of Artificial Intelligence (AI) have recently taken the world by storm, with conversations centred on its positive impact across a broad range of industries, as well as the negative aspect of it potentially taking over jobs and eventually replacing humans. You have probably heard someone utter these increasingly ubiquitous words or read somewhere that “AI will replace humans, but people who use AI will replace those who don’t.”
Beyond the fear of AI’s much-touted dark side, it has demonstrated positive use cases in manufacturing, technology, banking, automotive, and education sectors. Its use is increasing daily as businesses seek to enhance their efficiency and productivity. Governments are also exploring ways to utilize AI, a key driver of Industry 4.0, to improve service delivery efficiency, ensure fair access to services, and enhance citizens’ overall experience.
As AI adoption heats up worldwide, and as early adopters derive benefits inherent in using the technology, recent rankings show that Africa’s capacity to fully embrace AI is lacking. The Oxford Insights Government AI Readiness Index 2022, which measures a country or organization’s capacity to effectively utilize AI technologies to drive economic growth, social development, and overall welfare, ranks Sub-Saharan Africa with the lowest score of 29.38 globally, with many African countries falling at the lower end of the ranking spectrum. The main challenges contributing to Africa’s low AI readiness rank stem from the lack of government initiatives, skills shortages, and most crucially, the availability of quality data, which are the engines of AI models.
advertisement
AI systems undergo training on specific datasets related to the subjects they address. The capabilities of the final AI engine are inseparably linked to the quality of the training dataset. However, organizations in Africa frequently encounter difficulties in providing their AI algorithms with the required quality or quantity of data. This challenge is primarily due to limited access to the necessary data and the insufficient volume of data that has not yet been generated.
For AI models to be accurate, reliable, and unbiased, they need to be trained on high-quality data that is complete, error-free, accurate, and relevant. Data quality is a challenge in Africa due to the way it is collected, stored, and processed. Most organizations and governments in Africa collect data manually or store it in formats that cannot be used in AI models. The timeliness, completeness, relevance, and consistency of data quality are in question. Data collected manually, in particular, may contain errors or be incomplete with missing values.
Another significant problem in Africa is data access. Governments and organizations tightly control their data, leading to its scarcity, despite AI systems requiring large quantities of high-quality data to perform effectively and at scale. The continent suffers from a lack of clear guidelines and policies regarding data ownership and access, hindering collaboration, data sharing, and the development of AI ecosystems. Although governments collect vast amounts of data across sectors, accessing it remains a challenge.
advertisement
Data handling also raises privacy and ethical concerns. Data protection and privacy are critical concerns in AI adoption. AI systems often rely on large amounts of personal and sensitive data to make accurate predictions or decisions. There is a risk of unauthorized access, misuse, or mishandling of this data, leading to privacy breaches. AI systems fed with openly accessed data may process personal data without individuals’ explicit consent or knowledge.
According to the IBM Global AI Adoption report, 60 per cent of companies are not developing ethical AI policies, 52 per cent are not safeguarding data privacy throughout the entire lifecycle, 59 per cent are not guarding against adversarial threats and potential incursions to maintain system health, and 60 per cent are not tracking data governance changes in data and model versions. Addressing privacy and ethical concerns in AI requires a multidisciplinary approach involving policymakers, technologists, ethicists, and society.