advertisement
What is decision science? Transforming decision-making with data
One of the common aims of digital transformation is often phrased as “becoming a data-driven company.” Whether it’s business intelligence,…
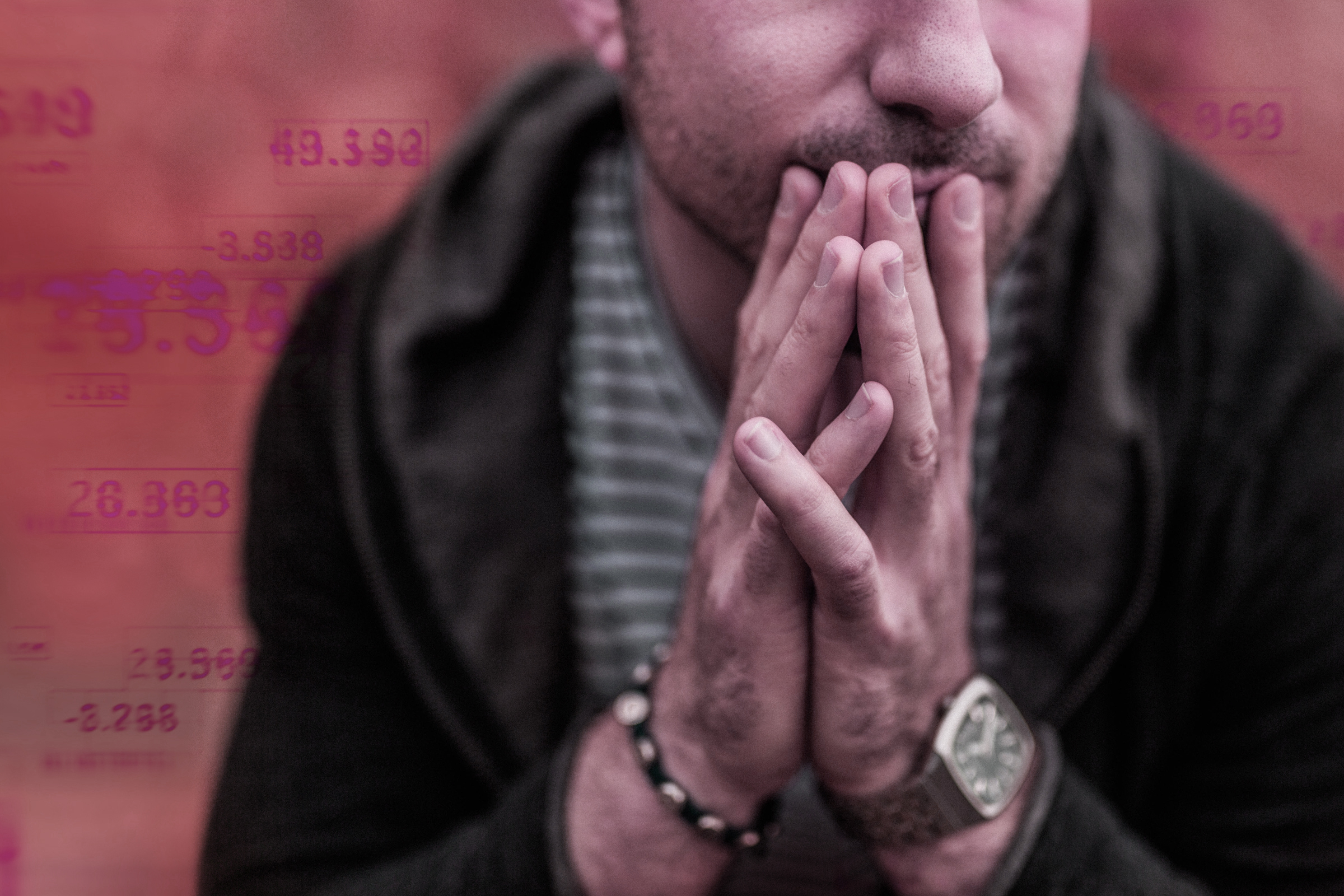
One of the common aims of digital transformation is often phrased as “becoming a data-driven company.” Whether it’s business intelligence, predictive analytics or machine learning, using objective data rather than hunches to drive decision-making is something businesses increasingly talk about.
There are plenty of challenges. Not all large organizations are nimble enough to make the most of data-driven decision making, or skilled enough to collect reliable data at scale and ask the right questions. A majority of executives in the annual NewVantage Partners big data study are looking to data to make better decisions, but only a third believe their company has a data-driven culture.
And data doesn’t have all the answers. Your data might not tell you that a successful product could be better, because the data says it’s already successful.
advertisement
Making data-driven decisions is about asking the right questions and having the right data to get the answers. You’ll start seeing that referred to as “decision science” (or “decision intelligence”).
What is decision science?
Google’s head of decision intelligence, Cassie Kozyrkov describes decision intelligence as combining tools and perspectives from disciplines that have previously been siloed and applying them to all aspects of selecting between options to reduce the effort required to make higher-quality decisions based on facts. “It brings together the best of applied data science, social science, and managerial science into a unified field that helps people use data to improve their lives, their businesses, and the world around them,” she writes.
There are similarities to fields like behavioral economics — which is about predicting market behavior and using incentives to change the market — and the intersection of computer science and economics known as EconCS, which Microsoft uses to design Bing ad auctions, Azure cloud pricing and Xbox game marketing.
advertisement
But decision science doesn’t have quite as broad a focus; it’s about solving specific business problems with data and algorithms by predicting the results of a decision.
Decision science vs. data science
This facet of predicting a decision’s results is a key differentiator between decision science and data science.
“Decision science is about using a mix of theory and data to predict what will happen after an individual or organization makes a decision that changes their environment,” explains Greg Lewis, senior principal researcher at Microsoft. It’s a crystal ball to help decision-makers find which is the right choice.
advertisement
Data science, on the other hand, predicts what will happen next if you don’t do anything to change the system, Lewis says. “For example, data science might be used to figure out that at the current rate, a store is going to run out of inventory; but decision science would help to decide whether it’s better to buy more inventory and keep prices the same, or raise prices so that the current inventory will be enough.”
Still, decision science is a part of data science, points out Chi-Yi Kuan, director of data science at LinkedIn, where until recently he ran the company’s decision science solutions team. He sums it up as “quantitative analysis and techniques used to inform and drive decision-making.”
“In data science we do a lot of work to understand what happened, what’s the history — that’s descriptive analytics. Then diagnostic analytics is drilling down and doing a deep dive to understand why it happened that way. When we get more advanced, we can do predictive analytics: What will happen in the future? What we call AI and machine learning in popular terminology is all about prediction and forecasting: Prescriptive analytics is about what would be the best option to have happen,” Kuan says.
Data science is about going from raw data to meaningful data insights; decision science applies that to recommendations for decision-makers. “The job of data science is [about] making better decisions in the ecosystem to help the company grow. In decision science, the focus is only on making the decisions,” he says.
Data-driven decisions for everyone
The principles of decision science should be familiar to executives because they’re already used in operational areas, but getting business insights from domain experts who give advice based on their experience (and limited amounts of data) is too slow and expensive to use for every decision. Decision science democratizes the ability to take advantage of real-time data, Kuan suggests. “We want to make it scalable. We want everyone at a company to be able to make data-driven decisions: It’s not just for the executives — and that’s a big change.”
That means more than just having a data science team; it means giving people the authority to make decisions with data. “A lot of companies have teams they call data science teams but they don’t give them authority and they don’t trust them to make recommendations,” Kuan says.
Data, he suggests, is useless unless you transform it into a decision. That means empowering people who have data they need to assess and a business problem they need to solve to use the data to help them make decisions.
Decision science examples
Kuan offers a long list of examples where decision science applies: A/B testing of products, marketing campaign performance evaluation, sales account lifecycle intelligence, and opportunities in customer service for improving customer experience.
The result might not be a single choice but a range of options: “In the digital world we can do a lot of A/B testing and make a decision on the results coming back,” he says. Almost every product decision at LinkedIn is shipped to a small set of users as an experiment, and product teams can see how those changes affect KPIs and other metrics.
LinkedIn applies decision science extensively, for everything from finding the right member segments for making email, to customer support, to using text analytics to understand member feedback, to the news feed — where it chose to make sure that everyday members get more exposure because comments and likes makes more of a difference to their engagement with the platform than they do to celebrities like Bill Gates and Richard Branson.
Employees do a lot of ad-hoc analyses, looking at what drives changes in specific business metrics; that’s all available through an analytics portal so the data science team can spend more time on analyses driven by questions and hypotheses, which help the business teams make their decisions.
Decision science is a journey
The data science isn’t done when the decision is made, Kuan points out. “After the decision making, the data science journey continues to measure what the impact of what we were working on and what we learn from the decision that was made, and keep improving that by experimentation,” he says.
That’s another big difference with decision science: “In the old way, when you made a decision it was basically done; you don’t have any opportunity to improve [in the future],” Kuan says. “Keeping track of the effects of those data-driven decisions gives companies an opportunity to learn from failure and grow the company.”
But decision science isn’t only for digital companies such as LinkedIn. What decision science gets you is a better decision-making process, Lewis explains.
“One of the best examples of decision science in practice is the rise of A/B experiments, where organizations systematically try out two different approaches and see which performs better with their customers,” he says. “A lot of the value added here is that running an A/B experiment forces teams to clarify what success looks like, what might be a good alternative to what they’re doing right now, and to put in place a process for continually updating their policies.”
That stops you from getting stuck — and you can still do it even if you can’t ship two versions of a product. “That’s when economics, game theory and causal machine learning based on existing customer data step forward to provide other ways of anticipating what might happen if a new direction is chosen,” Lewis says.
Getting to decision science
Decision science isn’t something you do instead of data science; it’s how you use data science to guide decision-making in your organization and it needs both data scientists and people who understand decision-making.
“Decision science is the application of the output of data science, merged with the context of stakeholder behavior and the business context of the problem,” Onepath CTO Patrick Kinsella told CIO.com. Implementing decision science takes more than adopting data science tools that can create models, draw conclusions and predict outcomes from data. “An organization must identify the team of individuals capable of assessing the behavioral impact of decisions. These individuals are typically experienced in change management through organizational transformation, merger/acquisition integration, or a redefinition of core values.”
Kuan emphasizes the importance of hiring for more than expertise in data science. Data scientists at LinkedIn need statistics expertise so they can design experiments and know how to measure if they’re successful. They need to know enough machine learning to formalize business problems as machine learning problems without mistaking correlation for causation. They need to know the business context for data well enough that they can interpret it, clean it and transform it into something usable. And they need to be able to present their findings and explain how they were created.
“The goal is to take the insights generated from the analysis and effectively influence critical decision-making, which drives business impact,” Kuan explains.
Lewis recommends teams that combine economists, statisticians and data scientists. “Economists figure out what needs to be measured to get a good sense of what’ll happen next; statisticians figure out how to measure it; and data scientists build the infrastructure and tools that allow regular and reliable measurements at scale.”
Don’t forget the basics either, says Miles Ward, CTO at SADA. “If you don’t have your data accessible, and easily queryable, with verified provenance and quality, making decisions off of it is going to prove haphazard. So if you’re not there yet, start on that.”