advertisement
GlobalData: AI is more important to IoT than Big Data insights
For enterprise software, operational efficiency trumps superfluous features. When it comes to optimising these applications, however, particularly for the Internet…
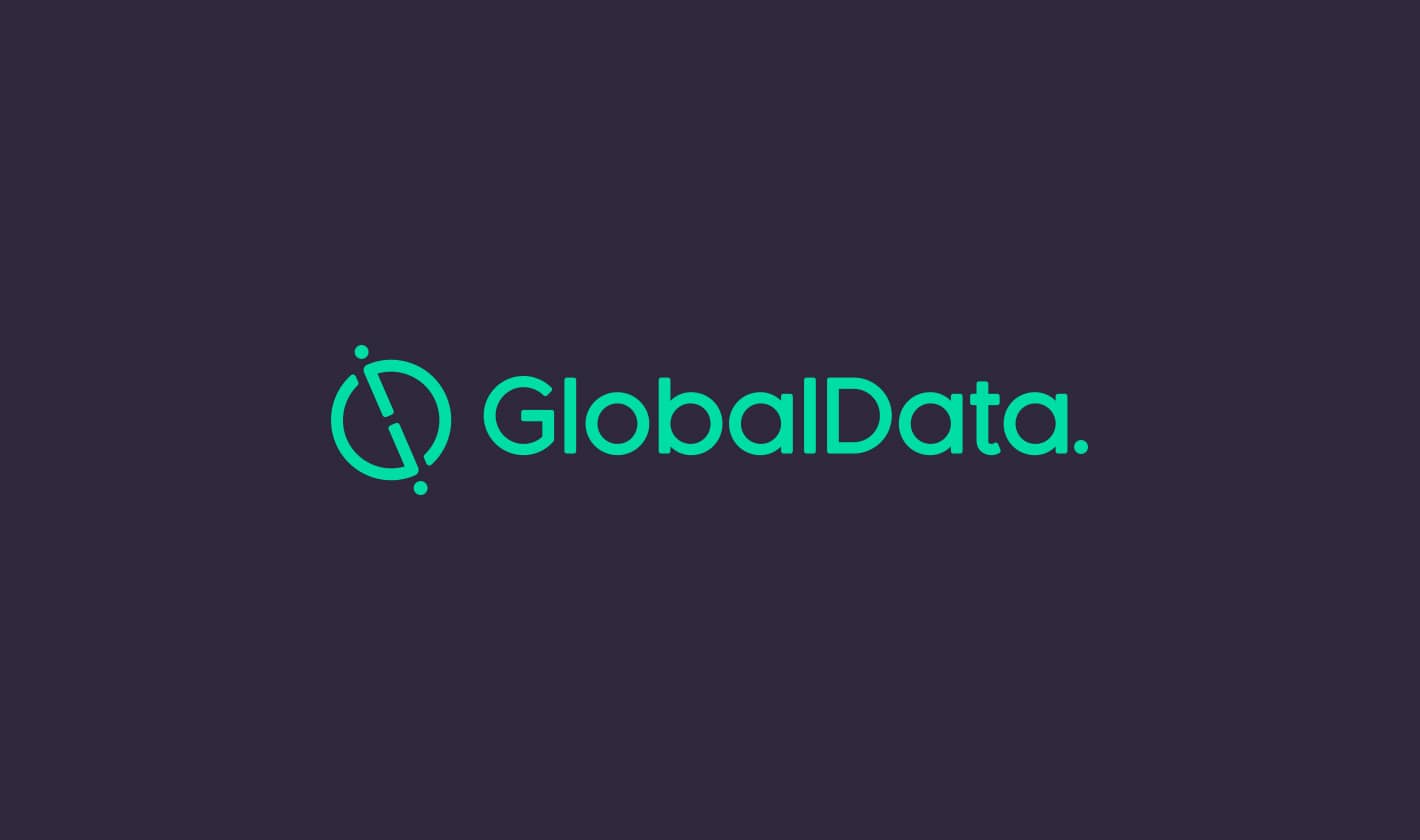
For enterprise software, operational efficiency trumps superfluous features. When it comes to optimising these applications, however, particularly for the Internet of Things (IoT), enterprises need to focus their efforts on the basics of business optimization rather than insight driven innovation.
A recent GlobalData survey of 1,000 IoT professionals revealed a heavy reliance on traditional business intelligence (BI) software.
40% of those surveyed ranked business intelligence platforms well above all other means of analysing data.
advertisement
Unfortunately, with the broad market trend toward the democratization of data now well-established, such do-it-all BI software platforms have already given way to numerous smaller, more discrete ways of deriving value from enterprise data, be that a direct SQL query, a predictive data modeller, an auto-generated data discovery visualisation, or a live, interactive executive dashboard.
The reasons for this are simple: business intelligence software is reactionary and static. Its users rely heavily upon basic reporting mechanisms that, in turn, rely heavily on laborious queries and reports – a very costly venture to both build and maintain.
This reluctance to follow the broader market away from BI platforms within IoT is concerning, given a subtle shift noted in the same survey concerning when, during its lifecycle, an IoT deployment fails.
advertisement
In 2016, no failures were noted post-deployment. In 2017, however, that number shot up to 12%.
“With deployment and maintenance costs also topping our survey as the number one reason IoT deployments fail or are abandoned prior to deployment,” noted Brad Shimmin, Service Director for Global IT Technology and Software at GlobalData.
“It becomes clear, therefore, that IoT practitioners should emphasize tactical benefits over strategic analytical insights at least at the outset of a project as a means of proving ROI and securing future investment from the business.”
advertisement
Artificial Intelligence (AI), however, can do far more than inform. It can immediately prove the value of IoT as a means of optimizing existing business processes.
With even the simplest AI machine learning (ML) framework and model at the ready, for example, IoT practitioners can solve two pressing problems: detecting anomalies and predicting desired outcomes.
GlobalData’s survey (see figure 1) shows that enterprise buyers are eager to do just that with 43% indicating that the best role for AI is to centrally automate and optimise business processes.
The problem lies within the idea of centralisation. Centralization is part and parcel to traditional BI analysis and reporting and traditional ideas like predictive modeling. Where AI is most vauable, however is at the edge.
IoT deployments need to employ tools like ML, not centrally, but at the edge, close to the device itself. And like today’s enterprise software, those analytics endeavours should be brief and to the point, and focused on solving specific challenges.
This is the same idea espoused by some branches of AI itself such as deep learning (DL), where the combination of numerous, smaller decision-making algorithms can give rise to a larger, seemingly intelligent system.
The idea is simple — avoid building an expensive monolithic analytics system centrally. Our advice, therefore, is that IoT buyers not only seek centralized, global visibility of the business but also local optimization via discrete, AI-driven outcomes.
This approach will not solve the full set of potential problems, but it is affordable and will have a direct and immediate impact on businesses, helping to prove the value of IoT one problem at a time.