advertisement
3 Enterprise AI Success Stories
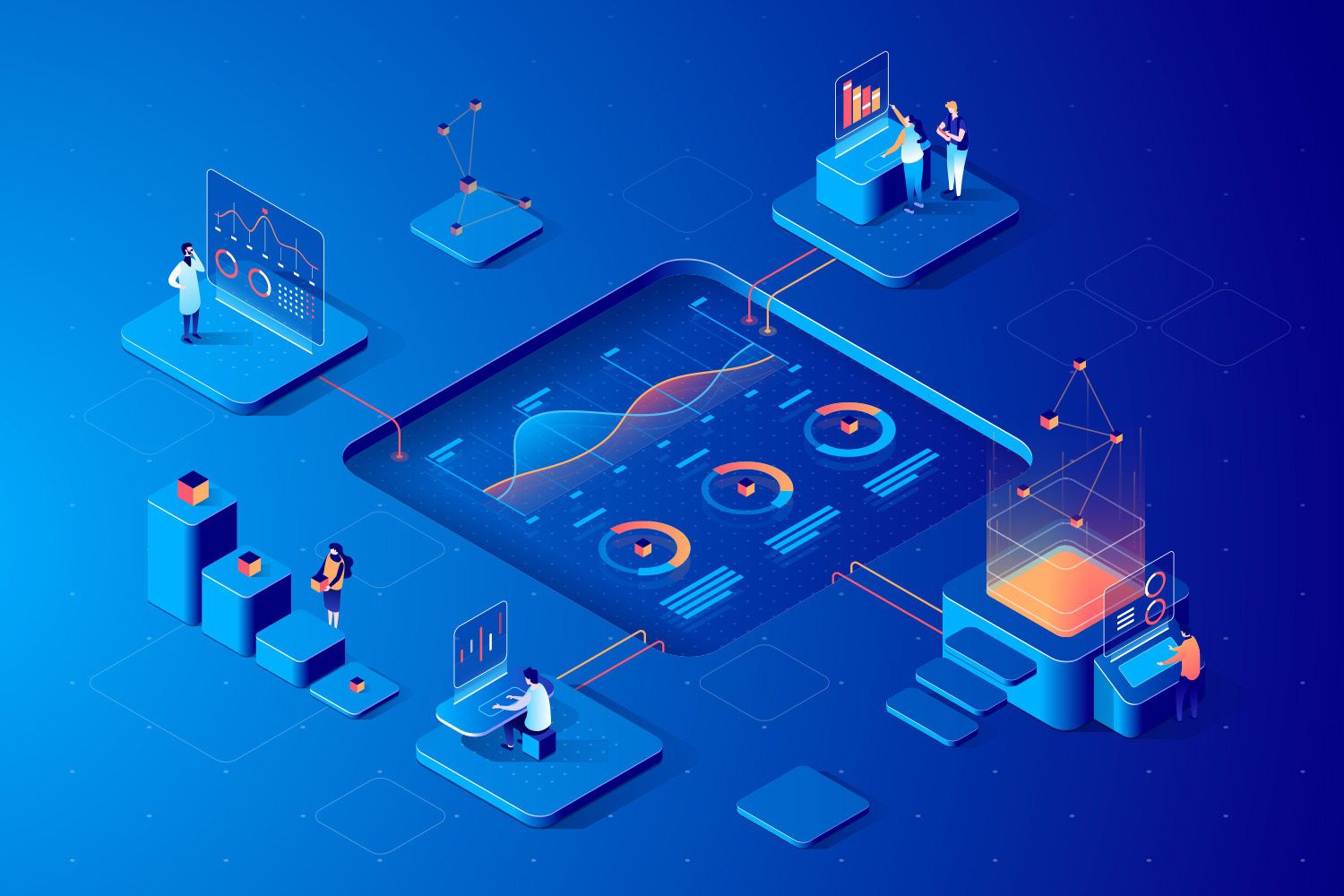
Artificial intelligence (AI) and machine learning (ML) might be high in the hype cycle at the moment. But that doesn’t mean organizations are not realizing tangible gains from deploying products that leverage the technologies.
Here are three examples of how AI and ML are improving internal business processes and paying off for enterprises.
Boosts for sales and marketing
advertisement
Beacon Street Services needed to have a “single source of truth” for all its company’s data, to ensure consistency and accuracy across its applications. The company is the services arm of Stansberry Holdings, which produces financial publications exclusively through purchased subscriptions.
Having collected and stored massive volumes of data using Snowflake, the cloud-based data warehouse service, Beacon Street Services wanted to use that data to help its sales and marketing teams improve on previous tactics and processes of selling subscriptions.
“Our marketing and sales teams saw an opportunity to improve on sales processes by applying a data science approach,” says David Kline, vice president of engineering at Beacon Street Services. “With this approach, we hoped to better identify buying criteria to help the marketing team run more effective campaigns.”
advertisement
Taking the historical user data the company had in its Snowflake data warehouse and loading it into an enterprise AI platform it deployed from DataRobot beginning in 2019, it was able to build a series of models quickly and automatically, using dozens of the latest data science algorithms. With these models, it identified buying criteria to help the marketing team run more targeted and effective campaigns.
The company now continues to feed large amounts of data into the AI platform from the data warehouse, Kline says.
As a result of the new process, Beacon Street Services saw a 10% increase in sales and is on track to realize $15 million in additional annual sales directly attributable to the AI platform. Since implementing the platform, the company has seen 30 to 35 times return on investment in revenue gains and cost decreases, Kline says.
advertisement
“For example, for one individual project we had to manually go through previous transactions to determine the risk of chargebacks following automatic subscription renewal and create a risk evaluation model, Kline says. “Not only was this process automated using AI, but we now have the benefit of proactively handling upcoming transactions.”
In addition to seeing improved accuracy and optimized marketing campaigns using AI, the DataRobot platform also provided significant time savings. Previously, it would take as long as six weeks to develop a model, with no guarantees that the optimal algorithm was selected. With the enterprise AI platform, that time to develop and deploy models that used more appropriate algorithms was reduced to just one week.
A side benefit is that the company’s IT team is spending less time analyzing data and more time working on potentially valuable projects for the business.
Classifying documents for better security
Company Nurse, which provides Covid-19 health screenings, workplace injury reporting, and nurse triage services for employers, is leveraging AI on several fronts.
One project involves enhancing the process of classifying documents. Company Nurse in 2020 deployed a platform from Concentric called Semantic Intelligence, to protect private workers’ compensation data on behalf of its customers and their end users.
The system autonomously discovers Company Nurse’s critical unstructured data, providing an opportunity to mitigate data sprawl and reduce threat surfaces.
As part of its service to customers, Company Nurse completes incident reports for workers’ compensation, providing appropriate care advice to injured workers and managing providers for referral. The information in the reports and forms includes significant amounts of unstructured data, says Henry Svendblad, CTO at the company.
By using the AI-powered system from Concentric, Company Nurse can protect private information in the documents without the need for staff to manually go through the data. The platform automates unstructured data security using deep learning to categorize data, uncover business criticality, and reduce risk.
Semantec Intelligence uses the baseline security practices seen for each category of data to calculate a “risk distance” from the baseline for each individual document. The risk distance uncovers events such as inappropriate sharing of information, risky storage locations, and incorrect classifications.
Not long after deploying the platform, Company Nurse was able to identify duplicate files it didn’t need to maintain, and found opportunities to enhance access permissions, Svendblad said. The company also found a large cache of private injury reports that were left on an open share application due to oversharing and lax access permissions.
“Any leakage of these documents would have been devastating to Company Nurse,” Svendblad says. “We have closed several small ‘blindspots,’ where files have been overshared, thanks to [AI] automatically classifying these documents for us. Preventing a single breach is worth tens of thousands [of dollars] to us. The cost of the solution is well worth the ROI—even if not a ‘hard’ ROI.”
In addition to the document classification process, Company Nurse is also applying AI-powered software products to functions such as sales opportunity scoring, data analytics, identification of zero day security threats, and speech-to-text transcription.
It’s difficult to estimate the investments the company has made in AI technologies because the tools are built into various software products it’s using, Svendblad says. But the company has seen multiple benefits, including improved security, enhanced work/life balance for employees, and improved quality of service in its contact center.
A step toward autonomous oil drilling
Oil and gas companies are under growing pressure to increase the efficiency of their drilling operations. One such company, Devon Energy, is in the early stages of leveraging AI and ML capabilities for real-time decision making that could potentially lead to closed-loop automation capabilities—or an autonomous drilling rig at each well site that would help to boost overall operations, efficiencies, and safety at the sites.
Devon Energy, which recently merged with WPX Energy (the company that initiated the push to AI/ML beginning in 2020), is using an offering called Hivecell to deliver edge computing at its drilling sites on oil fields in West Texas and North Dakota. Hivecell, provided by a company of the same name, is an “edge-as-service” offering that enables computing and analytics close to the source of the data.
The data is created by sensors in the equipment provided by a service company that Devon Energy uses for hydraulic fracturing processes. The data is transferred from the sensors to the service company’s systems and then is sent from those systems to Hivecell via the TCP/IP protocol.
Devon Energy is in the first phase of leveraging Hivecell to process machine learning at the remote well sites. Having this capability will enable Devon to avoid sending all of the data to the cloud for processing, which is costly and slow. Hivecell is used in conjunction with Confluent’s event streaming platform that manages the raw drilling site data from Apache Kafka.
“We’re always searching for ways to improve our drilling and completion operations efficiency,” and are looking to automate manual tasks through ML, says Dingzhou Cao, senior data science advisor at Devon Energy. The first phase of the project involves gathering real-time data on hydraulic fracturing, a well-stimulation technique involving the fracturing of bedrock formations by a pressurized liquid, so that natural gas and petroleum can flow more freely.
“We are in the process of building models to detect the hydraulic fracture events from real-time data automatically,” Cao says. “We wanted to be forward-thinking and are always looking for ways to build efficiencies and improve processes.”
Typically, oil and gas companies today rely on cloud computing services for processing and analyzing data from remote locations in near real time, Cao says, but this has the disadvantage of internet connection instability and latency issues.
“Think of a self-driving car that is processing data in the cloud,” Cao says. “Every millisecond counts when it comes to the vehicle reacting, to ensure the safety of the passengers and those around it. So, the amount of time it takes to send the data from the car to the cloud is crucial and could lead to safety incidents if it’s not in true real time.”
The same is true for closed-loop optimization; for example, auto-drilling rigs at the well sites. “We need to be able to react in real time, and can’t risk data being lost due to an unstable internet connection,” Cao says.
Devon Energy is building the foundation for true real-time, analytics-driven decision making, and eventually closed-loop control of the well sites. This will eventually allow the company to use edge-based stream processing for real-time, closed-loop control over drilling operations. The data streams produced by the machine learning models and analytical preprocessed data at the well site will also be replicated to the cloud for other purposes.
“Given that Hivecell runs on-site, we don’t have to worry about latency or internet instability issues,” Cao says. The platform will allow Devon to easily deploy, manage, and scale ML models at the well sites, he says.
Devon Energy hopes to complete the first phase in 2021 and then move to the second phase, in which it will determine the analytics models based on the most crucial business needs.
Although Cao did not provide specific cost information, he says the implementation is cost-effective compared with using a cloud-based option. “By leveraging Hivecell, we’re able to process the data on the stack and don’t need to pay the software licensing fee for a similar stream processing engine,” he says.
With the implementation, “we’re putting real-time data in the hands of who needs it most, when they need it most,” Cao says. “Our engineers have the ability to access the real-time data via their cell phones or computer. While we’re still in the first phase, which is just focused on capturing this data, as we move through future phases the analytics model will be used to aid well site decision making.”