advertisement
Machine learning in cybersecurity: what is it and what do you need to know?
Recent breakthroughs in machine learning and artificial intelligence mean AI-enabled technologies are gaining traction. The billion-dollar cybersecurity industry is no…
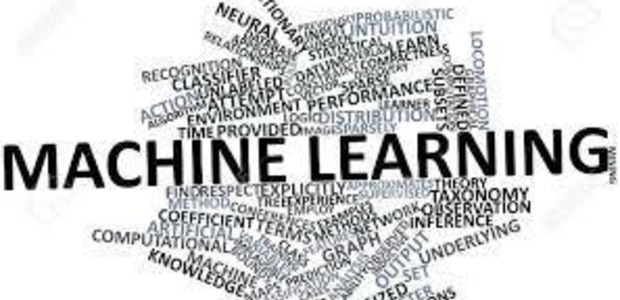
Recent breakthroughs in machine learning and artificial intelligence mean AI-enabled technologies are gaining traction. The billion-dollar cybersecurity industry is no exception, as vendors begin to scale and automate their processes intelligently – all while locked into the early stages of a security arms race with professional hackers.
A recent report from analyst firm ABI Research estimates that machine learning in cybersecurity will enormously bolster spending in big data, intelligence and analytics, reaching as much as $96 billion (£71.9 billion) by 2021.
Vendors are likely to find buyers in large enterprises, and more than likely, across industries that are especially prone to attack: think government and defence, banking, and across the technology sector. At the moment, ABI’s report says, User and Entity Behavioural Analytics – using machine learning for threat detection by analysing data at scale – is the driving force.
advertisement
“Using static machine learning models to detect previously unknown malware is the only use case I’m aware of that offers clear evidence of effective results,” says cybersecurity analyst at 451 Research, Adrian Sanabria.
“Most machine learning use in the industry right now is experimentation and seeing what sticks,” Sanabria says. “The fact that machine learning has had some success in one area of infosec practically guarantees that this industry will attempt to use machine learning anywhere and everywhere it can be shoehorned in.”
But threat detection is not a trivial matter: in Cisco’s recent annual cybersecurity report, it noted that the vast majority of companies are working to improve their threat detection capabilities.
advertisement
See also: Why machine learning could be the next frontier for data centre operations
There are plenty of public breaches where not only was the organisation unaware of the intrusion until it was far too late, they had no idea about the true extent of the breach. A case in point is the devastating Yahoo hack – where eventually the company discovered 1 billion email account details were compromised.
The author of the ABI paper, Dimitrios Pavlakis, tells Computerworld UK that to understand why machine learning is useful for detection, it’s important to define the two primary distinctions for machine learning.
advertisement
Supervised applications of machine learning tend to mean that you have clean and structured data – for example, anything that could be read in Excel – where you treat the model with what you know and what you then expect the software to do. In this case you tell the algorithm what to do and where to look.
But unsupervised applications can examine unstructured data from multiple data sources. “With unsupervised models in machine learning you can then teach a model using neural networks and deep learning,” says Pavlakis. “You can teach a machine learning algorithm to detect the unknown. The algorithms are being trained, models are being stacked and trained all together.
“So you feed them data and tell them this could be normal, for example, and if something strange happens, the unseen threat can then be flagged.”
Some cybersecurity vendors in the machine learning space include Splunk, Gurucul, and Vectra, Trend Micro, Symantec, Invincea, and CrowdStrike, and giant enterprises like IBM are also doing work in the field.