advertisement
How AI is revolutionizing financial services
Financial services firms are helping lead the way in adopting artificial intelligence, with banks expected to spend $5.6 billion on…
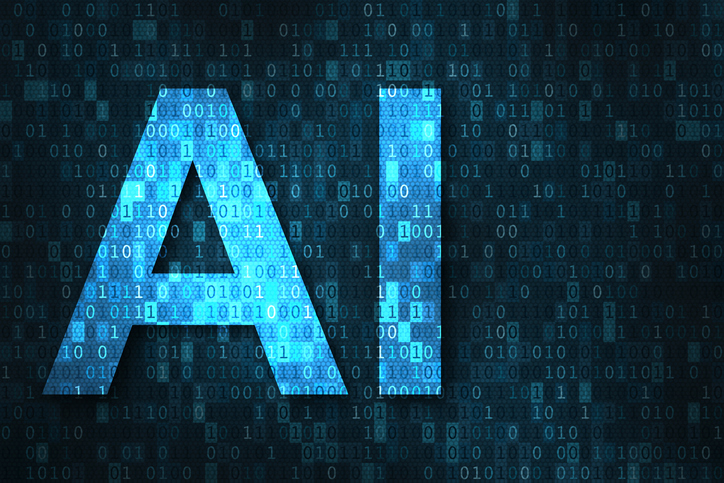
Financial services firms are helping lead the way in adopting artificial intelligence, with banks expected to spend $5.6 billion on AI solutions in 2019, second only to the retail sector, according to IDC.
And the fruits for finservs could be considerable: McKinsey Global Institute predicts AI and machine learning could generate more than $250 billion in value for the banking industry.
CIO East Africa will be hosting the East Africa Fintech Summit from the 22nd- 23rd of May 2019, click here to register for the event and learn more about Fintech.
Still, many financial firms remain cautious on artificial intelligence, thanks to potential financial, reputational, and regulatory fallout. Here, gaining greater insights into how AI systems arrive at decisions is key.
advertisement
In the meantime, financial services companies seeking competitive advantage are rolling out AI systems to support customer service operations, undertake risk analysis, and overhaul marketing and sales processes. Following is a look at how several financial services firms are putting AI to work.
Streamlining customer service
Synchrony runs credit cards for many major brands, including Gap and Old Navy, Amazon, JC Penney, Lowe’s, Sam’s Club, and American Eagle, servicing more than 80 million active consumer accounts. That’s a lot of customers who might need help with their cards, such as reporting fraudulent transactions.
Two years ago, the company went all-in on artificial intelligence and has already hired more than 170 data scientists, all while launching an emerging technology center at the University of Illinois. Like many financial services companies, Synchrony’s key deployment of AI and machine learning is in chatbots.
advertisement
“Our intelligent virtual agent, Sydney, resides on the majority of our retailers websites, including Gap and Lowe’s,” says Greg Simpson, the company’s CTO and AI leader. “If you had a question about your credit card with one of those accounts, you could ask Sydney, and Sydney would help answer basic questions.”
The platform currently handles half a million chats a month, drawing from answers based on years of calls to Synchrony’s call center. The platform, which is also available via Amazon devices, has helped cut live chat volume by more than 50 percent, Simpson says, and 88 percent of customers who used Sydney say they were satisfied with the service.
Sumitomo Mitsui Banking Corp., a global financial company and the second largest bank in Japan by assets, is similarly deploying AI for customer service. The bank uses IBM Watson to monitor call center conversations and automatically recognize questions and prompt operators with answers, thereby reducing the cost of each call by 60 cents. With more than a million calls a year, that’s $100,000 in annual savings, says Tomohiro Oka, a director at the bank. In addition, customer satisfaction went up by 8.4 percentage points, he adds.
advertisement
Oka moved to Silicon Valley in 2015 to run SMBC’s innovation office, and has headed up several AI projects at the bank.
“We are also using IBM Watson for our employee-facing interactions,” he says. “For example, if a sales person has a question about an internal rule and ask HQ in Japan, there’s a big time difference and the answer will be delayed by a day.”
Watson is used to automatically answer these questions, he says.
In the past couple of years, all the major banks have had chatbot projects in the works, says Gartner analyst Moutusi Sau. “There are many technologies for that,” she says. “Conversational chat engines, virtual customer assistants. They’re a big part of the pie.”
Banks are continuing to invest in the area, but the intelligent agents are now being put to work on improving internal operational efficiencies as well, she says.
Bringing intelligence to the sales process
One bank that decided to wait on chatbots was NBKC Bank, a midsize bank based in Kansas. Instead, NBKC is using AI as part of its mortgage lending process.
“Most of the AI you see in the mortgage universe is geared around chatbots for customer service,” says Chad Cronk, the bank’s EVP and director of mortgage. “We thought about it, but we think that area needs to grow quite a bit.”
At NBKC, AI helps distribute leads to loan officers. About 60 percent of new leads come in through online lead aggregators, such as Lending Tree and Zillow, averaging 300 to 350 new leads a day — the rest come from referrals and repeat customers. Previously, leads were distributed to the company’s 98 loan officers via a “round robin” system, says Cronk.
But in analyzing historical data, NBKC found that some loan officers were better at handling new leads early in the morning, say, or late in the afternoon, or had better success with customers from a particular geographical area.
“This led to the concept of distributing leads on an intelligent level,” says Cronk. “We thought if we paired prospects with the right officers at the right time, we would keep delivering a better customer experience.”
Due to its smaller size, the bank went with an outside vendor, ProPair, instead of building the technology in-house. ProPari’s platform has helped NBKC increase close rates by 10 percent, and improved performance of 65 percent of its loan officers.
CIO East Africa will be hosting the East Africa Fintech Summit from the 22nd- 23rd of May 2019, click here to register for the event and learn more about Fintech.
Today, 25 percent of leads go to a control group, assigned at random. The rest are assigned based on the intelligent system, which distributes leads to the best suited agent, taking into account individual workloads to ensure everyone is still receiving the same total number of leads.
“We’ve seen noticeable improvement,” says Cronk. “There were some quarters where the increase was 15 percent.”
Rolling out the new technology took around three or four months, he says. Data on leads from third-party aggregators comes in via APIs to the bank’s lead management system, Velocify. It took a little bit of work to figure out how to fit the agent recommendations into Velocify, Cronk says, and to create a secure environment so that ProPaid could study agents’ historical performance.
Analyzing risk
Financial services firms have long used statistical models to evaluate risk — credit risk in lending, financial risks in trading, actuarial risk in the insurance sector, as well as the risk of fraud across all categories.
“What’s different these days is that the use of these algorithms is much more extensive and the amount of data available, the types of data, and the throughput of data is changing the kinds of problems that are being solved,” says Chris Feeney, president of BITS, the technology policy division of the Bank Policy Institute. “If you can collect more information about transactions, you can do a better job to avert fraud.”
Feeney expects AI to become a big differentiator for financial firms. “You have to be active, but you have to pick your use case,” he says, suggesting firms look for opportunities to use AI to create a competitive edge, but also provide clear value to the consumer.
“It could be in the lending business,” he says. “There is a lot of activity now about using alternative sources of data to offer lending products to new groups of people.”
Fraud analysis is another important use case, he says. “I think AI is going to accelerate the ability to spot fraud faster in order to avert it, to spot anomalous activity faster.”
Raghav Nyapati agrees. “Consider underwriting,” says Nyapati, who recently led AI projects at a top-ten global bank and is now launching a financial technology startup. “We have thousands of applications coming in. AI can help filter out applications which may be fraudulent or high risk, and only the filtered ones are reviewed by an agent.”
CIO East Africa will be hosting the East Africa Fintech Summit from the 22nd- 23rd of May 2019, click here to register for the event and learn more about Fintech.
These decisions need to be backed up by human judgment, he explains. “We have to be doing responsible AI. We have stakeholders to answer to, customers to answer to. And if anything goes wrong, the bank has to pay huge penalties.”
A recent Gartner survey shows that 46 percent of financial services firms use AI for fraud detection.
In the securities industry, firms are using machine learning in pre- and post-trade risk analysis, says Monica Summerville, head of fintech and European research at Tabb Group.
“It’s very compute-intensive to do the risk analysis in a traditional way, and a lot of machine learning techniques, while approximations, are good enough and faster,” she says.
In a recent survey Tabb Group conducted, the majority of securities firms plan to expand spending on AI in the next 12 months. “It’s ranked as the most disruptive technology to their business,” she says.
According to Gartner, AI will impact more complex tasks as well, such as financial contract review or deal origination. The research firm predicts that by 2020, 20 percent of back-office staff will rely on AI for non-routine work.
Compliance challenges
Regulators are already familiar with the difficulties of overseeing the models used by financial institutions for, say, evaluating credit risk or spotting suspicious behaviors. The models might be highly complex, for example, and hard to analyze. Or they may be proprietary models from third-party vendors.
There are ways to address these issues, such as having independent reviews of models and using compensatory controls such as circuit breakers. In some ways, AI-powered systems can be treated the same as traditional statistical models, but they also pose additional worries.
“For its part, AI is likely to present some challenges in the areas of opacity and explainability,” said Federal Reserve board member Lael Brainard in a speech late last fall. “Recognizing there are likely to be circumstances when using an AI tool is beneficial, even though it may be unexplainable or opaque, the AI tool should be subject to appropriate controls.”
That includes controls about how the tool is built, how it is used in practice, and around data quality and suitability, she says.
CIO East Africa will be hosting the East Africa Fintech Summit from the 22nd- 23rd of May 2019, click here to register for the event and learn more about Fintech.
Explainability — also known as the black-box problem — is a particular issue for AI systems. With traditional statistical models, data scientists manually pick factors critical to a particular decision or predictions, and decide how much weight to give to these factors. AI systems, however, can identify patterns that were previously unknown and hard to grasp. That makes it hard for banks to comply with regulators such as the Equal Credit Opportunity Act and the Fair Credit Reporting Act, which require them to explain the factors they use in making their decisions.
“Fortunately, AI itself may play a role in the solution,” Brainard adds. “The AI community is responding with important advances in developing ‘explainable’ AI tools with a focus on expanding consumer access to credit.”
The securities industry is also working on this issue, says Tabb’s Summerville. “Can you build an unbiased model in AI?” she asks. “You need to be able to explain how you made the decision. Regulators are interested in making sure you don’t introduce bias by accident.”
As Synchrony starts to look at AI and machine learning for credit decisions, the black box problem is becoming an issue for that company as well. “We’re looking to build explainability into our models, and point to the reasons why the decisions are made,” says Synchrony’s Simpson. “This isn’t easy to do.”
For example, he says, decisions can’t be made for discriminatory reasons. “You can’t say, ‘I won’t give credit for someone in this Zip code,’ because it’s illegal.”
The company is also spending a great deal of effort to make sure that the raw data used to train AI models isn’t biased. This is one of the reasons the company needs so many data scientists, Simpson says.
One approach the company is taking to reduce bias is to start with a diverse team.
“Without a diverse team, it’s hard to identify bias in your data because your team may be biased,” he says. “And it’s particularly important to us, being a bank. Diversity of your team is the first and best defense in this space.”
CIO East Africa will be hosting the East Africa Fintech Summit from the 22nd- 23rd of May 2019, click here to register for the event and learn more about Fintech.