advertisement
AI: A Force For Good In Curbing COVID-19
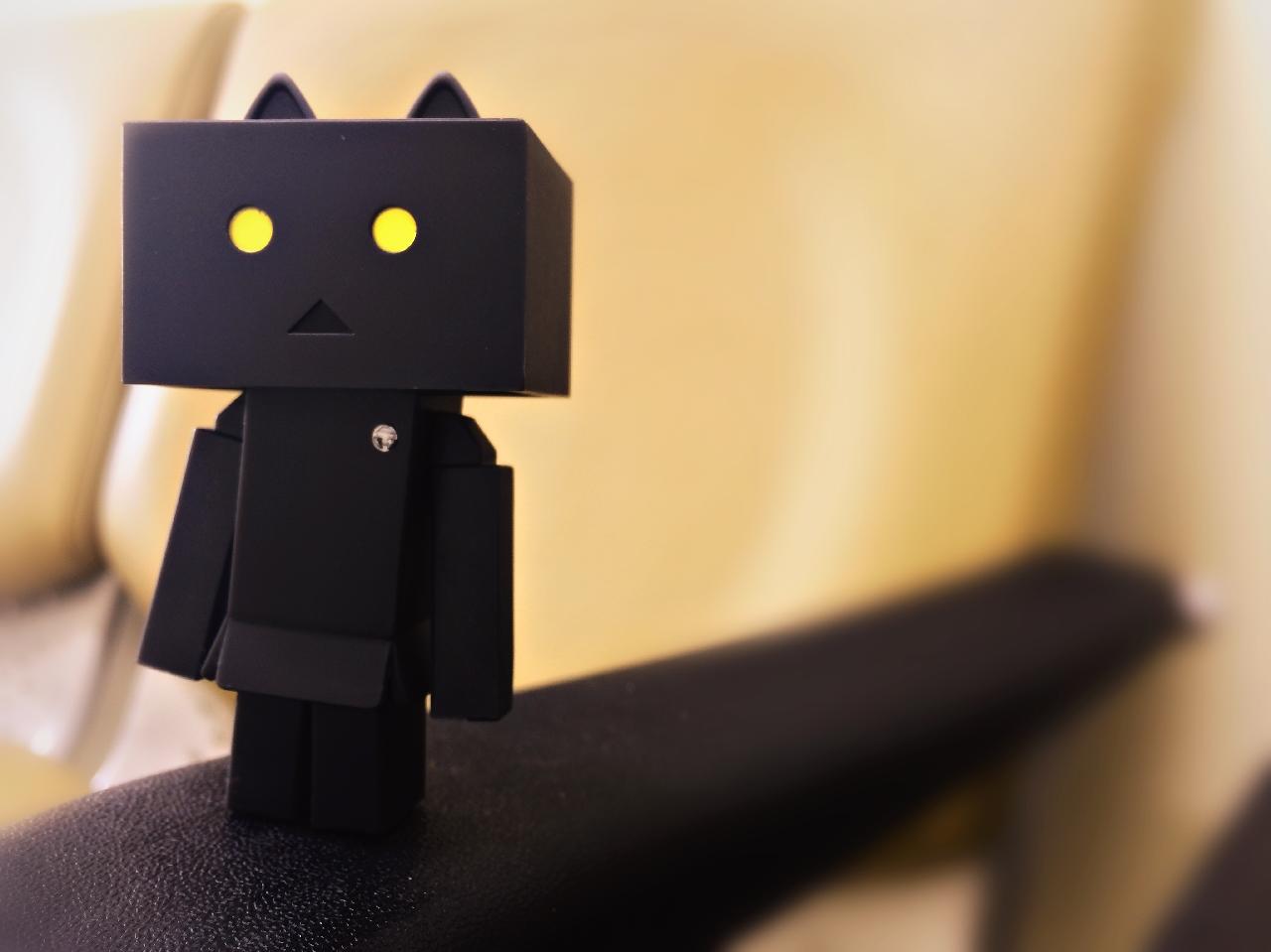
It was John McCarthy, recognised as one of, if not the father of artificial intelligence (AI), who defined it as “the ability of a machine or a computer to think and to learn.” With AI coined in Dartmouth Campus in 1956, it would be decades before technology, aka computing eventually caught up with his genius. Now, AI captures both beauty of complexity and complexity logic. In the webinar titled AI4G: How Artificial Intelligence Is And Can Assist In Curbing The Pandemic, the conversation turned to the concern du jour – COVID-19.
As one of the two presenters, Georgina Mukami Odhiambo, banker, a woman passionate about technology especially AI and a service delivery ambassador with over 12 years experience handling projects and evaluating the technology, reminds us how for a time there, it looked like AI might fail. It wasn’t until the world moved from robotics and begun pulling enough computing power. Back in the founding AI days, “there weren’t proper tools and frameworks then. Now, it goes a step further to allow AI as a service.”
Georgina can’t get enough of AI which “… has changed the way we live, work and relate. We work on devices with large amounts of transactions and process data. Now, the pandemic has changed everything AI did. It also brought about uncertainty in 4IR. We need to be able to harness data so we can become agile and churn out products. AI has never been more relevant.”
advertisement
She witnesses its use in the financial services industry (FSI) in back-office processes such as automation and repetitive tasks and processes, fraud detection and anti-money laundering. These churn a world of data to help in making better dnd fair credit decisions to determine someone’s credit score, chatbots “… though AI zealots may not consider them native to AI, but they mimic humans. They can collect data for fundamental needs,” to alternative data for algorithmic training to get correct pricing and performance metrics for decisions.
Enter COVID-19 where consumer lending, job loss, pay cuts, distress resulting from the lack of steady income – all existing factors but not to the unprecedented degree the pandemic has wrought – border on chaotic. How, for instance, can a bank determine who is in distress or likely to default because of pay cuts? That despite digital banking, there are people who still need to visit individual offices in person such as the National Hospital Insurance Fund (NHIF) and the Kenya Revenue Authority (KRA) despite social distancing and quarantine? With disrupted human contact functions and the workforce will be affected, the call centre team reduced and vulnerable employees who do not know if they will still have a job listening to CNN and BBC, to get information. The worst part, an absent mind is distant and unsure of itself, which leaves it an easy cyber fraud target.
How, for instance, can a bank determine who is in distress or likely to default because of pay cuts? That despite digital banking, there are people who still need to visit individual offices in person such as NHIF and KRA despite social distancing and quarantine?
By tapping into the 1965 AI objectives, it is evident AI as a service is on the right path. Let us keep in mind digital transformation plans have accelerated. Whatever, Georgina says “was pegged for 2023 is happening now. Uncertainty affects what you know about survival in as short a time as three months. Virtual assistants who handle accounts and personal financial management, credit decisions assessment for informed, fair decisions, decisions around fraudulent transactions using predictive models will all shift.”
advertisement
Like AI, the pandemic has changed how we live, and brought about uncertainty, and allows for one to quickly adopt. But, how do we harness data fast so that we can use AI? Both have upended FSI and the banking industry. “We knew about AI all along, but it just became a reality. We have been forced to move it ahead. These are things we knew but are now becoming a reality making virtual assistants critical. They will feed from data.” No industry can afford reduced customer satisfaction levels. Yet they must deal with trigger-primed employees expecting everything is bad news. AI can identify the pattern, protect you all while curbing the pandemic using predictive data for yourself and employees.
With a background in technology and business management, John Kamara, founder Afya Rekod; director for Machine Intelligence Institute of Africa MIIA, Cape Town; Director for Innovation Nelson Mandela African Institute of Science and Technology (NM-AIST), Arusha and founder Adalabsafrica among other things, John sees it all In sharp relief.
AI does not work without data; neither does artificial modelling. Think tabula rasa. It raises the question of ethical AI. Data sets will always give back what you put in. Machines do what they are built to do. There is no reason for the data to do anything other than what it was made and intended for. Machine-learning tools such as Sophia the Robot learn in nanoseconds, uses this information to run multiple variables, but it is still information she gets from the world. It cannot be emphasised enough that the data you put in is the data you get out. Should this not be the case, John says, then the “problem is in the human error, less the machine error.” His solution is educating the young generation about ethical AI.
advertisement
Machine-learning tools such as Sophia the Robot learn in nanoseconds, uses this information to run multiple variables, but it is still information she gets from the world. It cannot be emphasised enough that the data you put in is the data you get out.
A more common example of machine-learning is Netflix, helping you make decisions better by using conversational data which is influenced by the emotional management of your choices. Thank that for your unique recommendations.
There are multiple different versions of AI.
- Pure AI: use of datasets for predictive intelligence, decision making, precision or dynamic information or pricing (which he did with a South African bank when working in insurance, scaling it by 28%)
- Conversational ai like chatbots: learn and mimic the interaction of the consumer beginning to create value.
- Self-learning machines: these are built based on neuro networks that allow machines to learn without being programmed. It becomes a neuro network on its with the ability to think, evolving into a quantum machine learning tool.
Consumer-driven sectors such as FS and healthcare are going to be led by AI if not yet, soon. Afya Rekod are building a consumer-driven healthcare platform. This allows them to look at disease correlation as a map. Look at consumer, their profile, data coming in through platform, and look st their potential risk with recommendations, and consumer intelligence is thus acquired before treating the patient.
It involves working with data sets from unstructured data to create a keyword correlation with data to show theirs is a higher risk of potentially a particular kind of disease when looking at keywords and correlation of disease to isolate individual needs. “In COVID-19, we are looking at the same information and then doing dynamic information modelling to establish if there is a high rate of potential COVID-19 cases in certain wards. We check if in said wards there are a lot of people with diabetes of HIV+ people. We then correlate and simulate what the disaster protocol might look like in that particular ward if you take in those two sets of data from live and active data, and testing data modules from the partners we are working with.”
This presents the opportunity to provide the data information that, and help counties predict potential outbreak areas and correlate health workers, community and map the information coming in. “We can then use it to pre-predict what happens and the movement of health workers within the communities and the propensity of that community to have a higher hit rate for COVID-19. We focus on the underbelly of this disease and the data and look at which one has the higher kill rate or kill value if you convert it with COVID-19. I call it Ebola-meets-COVID or HIV-meets-COVID data aside from just looking at where the outbreak may be,” says John.
We can then use it to pre-predict what happens and the movement of health workers within the communities and the propensity of that community to have a higher hit rate for COVID-19. We focus on the underbelly of this disease and the data and look at which one has the higher kill rate or kill value if you convert it with COVID-19.
Looking at healthcare, AI can allow the creation of 50 to 100 different variations of sequencing in the case of an operation and precision decision making in real-time very quickly. A decision that would have taken a doctor up to six months to make. With a brilliant learning tool with connectivity and cognitive disease modelling, you find that you can do this uber-fast with different variations and sequences. All that is left is just for the humans to make a decision on which one works best. “Now you have killed time, saved the patient’s life and the doctor’s time.”
Most organisations need basic AI to make quick decisions to increase ROI, sell better products, know who to sell it to, or efficiency. On that note, most companies with a data scientist and two engineers will allow you to build a fundamental model where you don’t need a large amount of computing power. That being said, the biggest problem for AI in Kenya:
- Data sets – The existing ones are not machine-readable. The question then becomes, do I build a machine first, or do I clean up the data sets? “That is where I tell a lot of clients to start. Let us look at the data, the objectives, then understand if the pair correlate, or if we need to create filter models. This helps understand what the data is saying, allowing us to build a module around it,” John points out.
- Are you using AI for conversational modelling around customer service, e-commerce, internet banking? You need simulator machine learning tools to mimic information it is receiving while creating multiple filters in real-time where it stores this information. And allow machines to talk to the customer consistently. It then prompts consumers and takes in data sets and merges it so you can understand your customers in fresh, different ways. It means you need to learn how to clean your data set, which takes a lot of time and money. Hence the need to create data sets that are machine-readable. “We see lots of banks with lots of data that is not machine-readable so they can’t use it,” says John.
“What I really enjoy is when you come to that dynamic and precision modelling of individuals. That is where you earn your dollar because you then know them at a more intimate level. This is what we do as an organisation. To use machine learning to find solutions in insurance and to do cognitive disease modelling at the same time,” he concludes.